Technology
Unraveling the Melody: Machine Learning Audio Fingerprinting
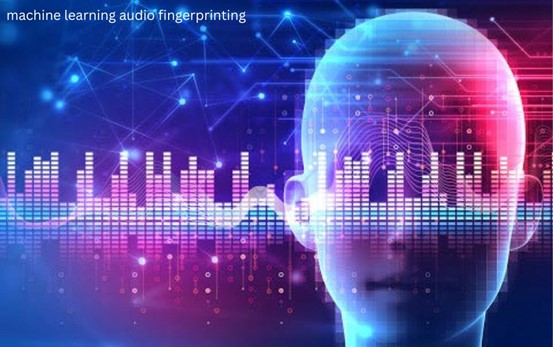
Introduction
In the digital age, where music permeates our lives, the ability to quickly and accurately identify a song has become a commonplace expectation. This is where machine learning audio fingerprinting, a technology that has revolutionized music recognition, comes into play. By analyzing the unique characteristics of a sound, audio fingerprinting can instantly match it to a vast database of songs, providing a seamless user experience.
Understanding Audio Fingerprinting
Audio fingerprinting, essentially, is the process of creating a unique, compact representation of a sound file. This representation, often referred to as a “fingerprint,” is generated by extracting distinctive features from the audio signal. These features could include:
- Frequency patterns: The specific frequencies present in the sound.
- Time-domain characteristics: The amplitude of the sound wave over time.
- Spectral envelope: The overall shape of the frequency spectrum.
Once the fingerprint is created, it is stored in a database. When a new audio sample is encountered, its fingerprint is calculated and compared to the existing database. If a match is found, the corresponding song or audio file is identified.
The Role of Machine Learning
Machine learning algorithms play a crucial role in audio fingerprinting. They are used to:
- Feature extraction: To automatically identify the most relevant features from the audio signal.
- Fingerprint generation: To create robust and efficient fingerprints that can withstand variations in audio quality.
- Database indexing: To organize and search the database efficiently.
Popular Machine Learning Techniques
Several machine learning techniques have been employed for audio fingerprinting, including:
- Hashing: This involves converting the audio features into a fixed-length hash value. Popular hashing algorithms include Locality-Sensitive Hashing (LSH) and MinHash.
- Neural networks: Deep neural networks, such as Convolutional Neural Networks (CNNs), can learn complex patterns in audio data and generate highly discriminative fingerprints.
- Support Vector Machines (SVMs): SVMs can be used to classify audio fingerprints based on their similarity to known fingerprints.
Applications of Audio Fingerprinting
Audio fingerprinting has found applications in various domains, including:
- Music recognition: Shazam, SoundHound, and other music recognition apps leverage audio fingerprinting to identify songs based on a short snippet.
- Content identification: Broadcast monitoring systems use audio fingerprinting to detect copyright infringement and track the usage of copyrighted content.
- Personalized recommendations: Music streaming platforms like Spotify and Apple Music use audio fingerprinting to create personalized recommendations based on users’ listening habits.
- Audio forensics: Law enforcement agencies can use audio fingerprinting to identify audio recordings, such as phone calls or surveillance footage.
Challenges and Future Directions
Despite its widespread use, audio fingerprinting still faces certain challenges:
- Robustness to noise and degradation: Audio quality can vary significantly due to factors like compression, noise, and channel distortions. Developing algorithms that are robust to these challenges is an ongoing area of research.
- Scalability: As the size of audio databases grows, efficient indexing and search algorithms become essential.
- Privacy concerns: The collection and storage of audio fingerprints raise privacy concerns, especially when used for surveillance purposes.
In the future, we can expect further advancements in audio fingerprinting, driven by advances in machine learning and deep learning techniques. New applications, such as real-time audio search and augmented reality experiences, are also likely to emerge.
Conclusion
Machine learning audio fingerprinting has transformed the way we interact with music and other audio content. By enabling rapid and accurate identification of sounds, this technology has opened up new possibilities in entertainment, content management, and law enforcement. As research continues to advance, we can anticipate even more innovative applications of audio fingerprinting in the years to come.
FAQs
1. How does audio fingerprinting work?
Audio fingerprinting involves extracting unique features from an audio signal and creating a compact representation (fingerprint) of the sound. This fingerprint is then compared to a database of known fingerprints to identify the corresponding song or audio file.
2. What are the key components of audio fingerprinting?
The key components of audio fingerprinting include:
- Feature extraction: Identifying distinctive features from the audio signal, such as frequency patterns, time-domain characteristics, and spectral envelope.
- Fingerprint generation: Creating a compact and robust representation of the audio signal based on the extracted features.
- Database indexing: Organizing and storing the fingerprints in a searchable database.
- Matching algorithm: Comparing the fingerprint of a new audio sample to the existing database to find a match.
3. What are the common machine learning techniques used for audio fingerprinting?
- Hashing: Converting audio features into a fixed-length hash value using algorithms like Locality-Sensitive Hashing (LSH) and MinHash.
- Neural networks: Deep neural networks, such as Convolutional Neural Networks (CNNs), can learn complex patterns in audio data and generate highly discriminative fingerprints.
- Support Vector Machines (SVMs): SVMs can classify audio fingerprints based on their similarity to known fingerprints.
4. What are the applications of audio fingerprinting?
- Music recognition: Shazam, SoundHound, and other music recognition apps use audio fingerprinting to identify songs.
- Content identification: Broadcast monitoring systems detect copyright infringement and track content usage.
- Personalized recommendations: Music streaming platforms create personalized recommendations.
- Audio forensics: Law enforcement agencies identify audio recordings.
5. What are the challenges in audio fingerprinting?
- Robustness to noise and degradation: Audio quality can vary due to factors like compression, noise, and channel distortions.
- Scalability: As databases grow, efficient indexing and search become essential.
- Privacy concerns: Collection and storage of audio fingerprints raise privacy concerns.
Technology
Why Are Aviator Gloves Essential? Purpose, Benefits, Best Picks
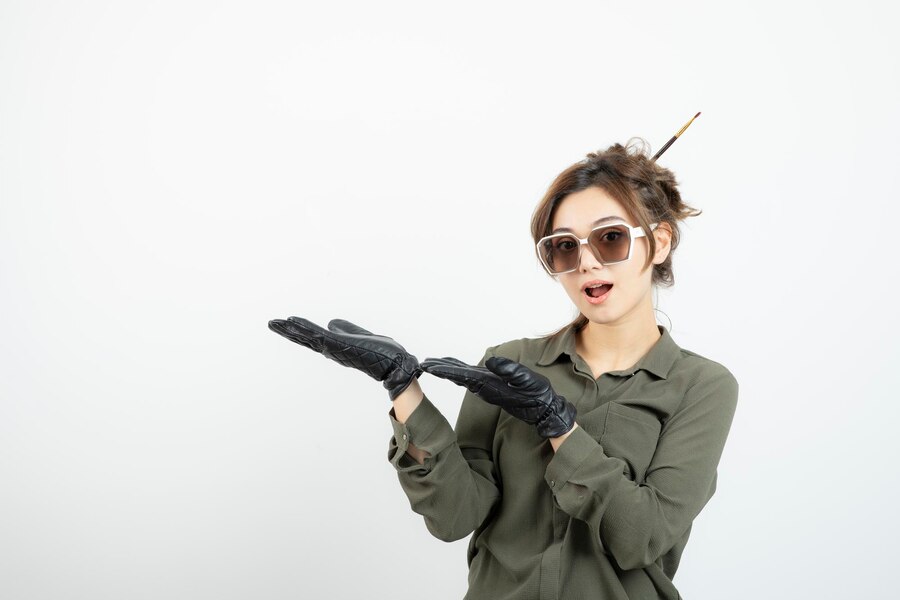
Introduction
Aviator gloves have long been an essential part of a pilot’s gear, but why are aviator gloves so crucial? These gloves serve multiple functions, from providing superior grip to protecting hands from extreme temperatures. Whether you’re a professional pilot, aviation enthusiast, or simply interested in high-quality gloves, this guide will explain their importance, features, and top recommendations.
What Are Aviator Gloves?
Aviator gloves are specialized handwear designed for pilots to offer protection, comfort, and enhanced control over aircraft instruments. They are crafted from durable materials such as Nomex, leather, and advanced synthetics to withstand harsh aviation environments.
Why Are Aviator Gloves Important?
1. Heat and Cold Resistance
Aviator gloves protect pilots from extreme temperatures, whether soaring at high altitudes or flying in frigid conditions. They help maintain hand dexterity in cold cockpits and prevent burns from hot controls.
2. Enhanced Grip and Control
The materials used in aviator gloves, especially leather and rubberized textures, provide a superior grip. This is crucial for handling flight controls with precision and avoiding slips during turbulence.
3. Protection from Fire Hazards
Many aviator gloves are made with flame-resistant materials like Nomex, which can protect hands from sudden cockpit fires or electrical malfunctions, increasing pilot safety.
4. Improved Comfort and Reduced Fatigue
Long flights require optimal hand comfort. Aviator gloves are ergonomically designed to reduce hand strain, ensuring pilots remain focused and comfortable throughout their journey.
5. Compliance with Aviation Standards
Aviation organizations, including the U.S. Air Force and FAA, require pilots to wear protective gear, including gloves, for safety reasons. Owning the right pair ensures compliance with these regulations.
Key Features to Look for in Aviator Gloves
When purchasing aviator gloves, consider the following factors:
✅ Material: Look for flame-resistant Nomex or high-quality leather for durability.
✅ Fit & Dexterity: Ensure a snug fit without restricting movement.
✅ Grip & Texture: Non-slip palm material helps maintain better control.
✅ Thermal Insulation: Adequate insulation for cold-weather flights.
✅ Touchscreen Compatibility: Useful for pilots using digital instruments or tablets.
Best Aviator Gloves in 2025
To help you choose, we’ve compiled a comparison table of the best aviator gloves available today:
Glove Model | Material | Best For | Pros | Price |
---|---|---|---|---|
Nomex Flight Gloves | Nomex & Leather | Military & Commercial Pilots | Fire-resistant, snug fit | $30-$50 |
Mechanix Wear Tactical Gloves | Synthetic Leather | General Aviation | Good grip, touchscreen-friendly | $25-$40 |
Wiley X Hybrid Gloves | Kevlar & Leather | Extreme Conditions | High durability, thermal insulation | $60-$80 |
Flyboys Pilot Gloves | Leather & Cotton | Private Pilots | Comfortable, lightweight | $20-$35 |
How to Maintain Aviator Gloves for Longevity
🧤 Cleaning: Use mild soap and lukewarm water; avoid harsh detergents.
🧤 Drying: Air-dry gloves naturally; avoid direct heat.
🧤 Storage: Keep gloves in a cool, dry place to prevent material degradation.
🧤 Regular Inspection: Check for wear and tear to ensure continued protection and performance.
Where to Buy Aviator Gloves?
You can find high-quality aviator gloves at:
- Online Retailers: Amazon, PilotMall, and Sporty’s Pilot Shop
- Aviation Gear Stores: Local flight gear shops and military surplus stores
- Manufacturer Websites: Direct from brands like Wiley X, Mechanix, and Nomex
Final Thoughts
So, why are aviator gloves an essential investment? They provide unmatched protection, enhance flight safety, and offer pilots the comfort and grip they need for precision control. Whether you’re a seasoned pilot or a beginner, choosing the right pair of gloves can make a significant difference in your aviation experience.
Technology
Discover the Latest Tech Trends on Zerodevice.net
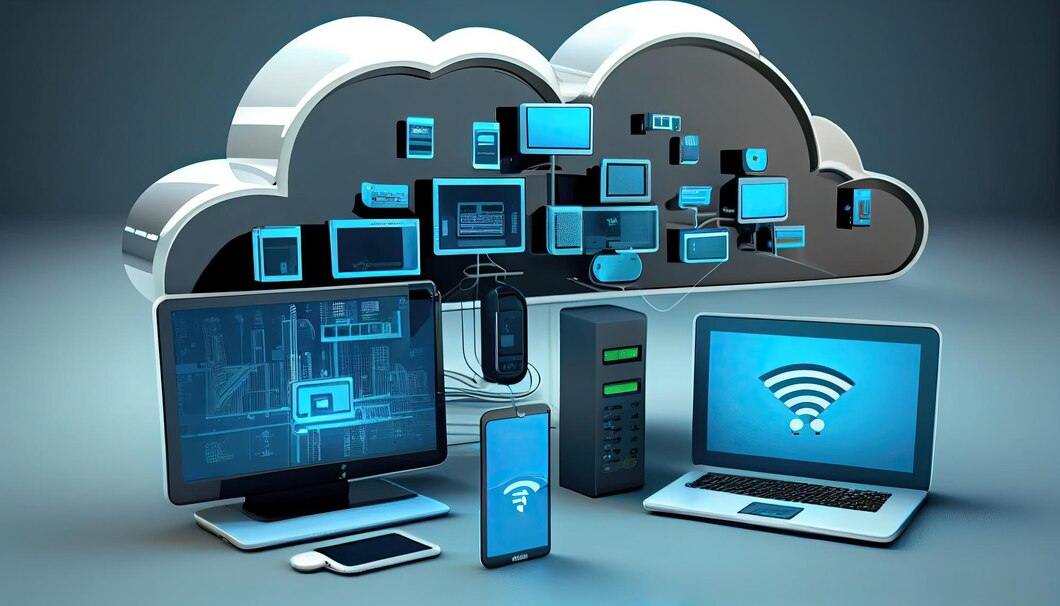
Welcome to the cutting-edge world of technology at Zerodevice.net, where innovation meets sophistication. Are you ready to dive into the realm of emerging tech trends that are shaping the future? Join us on this exciting journey as we explore revolutionary tools like Flipper Zero and groundbreaking networking technologies such as ZeroTier. Stay tuned as we uncover the latest advancements in tech and discover how they can elevate your digital experience to new heights!
Overview of Zerodevice.net
Are you ready to dive into the exciting world of technology? Look no further than Zerodevice.net, your go-to hub for the latest tech trends and innovations. This cutting-edge platform is a treasure trove of information for tech enthusiasts, offering in-depth insights into emerging technologies that are shaping our future.
Zerodevice.net is not just another tech website; it’s a community where like-minded individuals come together to explore the possibilities of tomorrow. From reviews on revolutionary gadgets to tutorials on groundbreaking software, this site covers it all. Whether you’re a seasoned tech pro or just starting your journey, Zerodevice.net has something for everyone.
With a user-friendly interface and constantly updated content, Zerodevice.net ensures that you stay ahead of the curve in the fast-paced world of technology. So why wait? Dive into Zerodevice.net today and unleash your inner tech enthusiast!
Emerging tech trends in the industry
The tech industry is always buzzing with new advancements and innovations that shape the way we interact with technology. From AI and machine learning to blockchain and cybersecurity, there are endless possibilities for growth and development in this fast-paced field.
One of the emerging trends making waves in the industry is wearable technology. Devices like smartwatches, fitness trackers, and AR glasses are becoming increasingly popular as they offer convenience and enhance our daily lives.
Another trend to watch out for is 5G technology, which promises faster data speeds, lower latency, and improved connectivity for a wide range of devices. This advancement will revolutionize how we communicate, work, and consume content on-the-go.
Cybersecurity remains a top priority as more data is being shared online than ever before. With an increasing number of cyber threats targeting individuals and businesses alike, investing in robust security measures has become crucial to safeguard sensitive information.
Flipper Zero: A Revolutionary Multi-tool
Are you ready to take your tech game to the next level? Introducing Flipper Zero – the revolutionary multi-tool that’s changing the game for tech enthusiasts everywhere. This compact device is packed with a variety of features that make it a must-have for anyone looking to streamline their digital lifestyle.
With Flipper Zero, you can say goodbye to carrying around multiple tools and devices. This all-in-one solution combines functions like universal RF remote control, contactless card emulator, cybersecurity tool, and more into one sleek package. It’s like having a Swiss Army knife for the digital age!
Not only is Flipper Zero incredibly versatile, but it also boasts top-notch security features to keep your data safe. Whether you’re managing access controls or testing network vulnerabilities, this device has got you covered. Plus, its user-friendly interface makes it easy for tech novices and experts alike to navigate.
Stay ahead of the curve with Flipper Zero – your go-to gadget for all things tech-related!
Introduction to Flipper Zero
Are you ready to dive into the world of cutting-edge technology? Let’s introduce you to Flipper Zero, a revolutionary multi-tool that is changing the game. This compact device packs a punch with its versatility and functionality.
Flipper Zero is designed to be your ultimate gadget companion, combining multiple tools in one sleek package. From hacking and pentesting to controlling devices remotely, this little powerhouse can do it all.
With features like RFID cloning, infrared control, NFC emulation, and more, Flipper Zero opens up a world of possibilities for tech enthusiasts and professionals alike. Its intuitive interface makes it easy to navigate through various functions seamlessly.
Whether you’re looking to enhance your cybersecurity skills or streamline your daily tasks, Flipper Zero is here to make your tech dreams a reality. Stay tuned as we delve deeper into the practical applications of this game-changing device on Zerodevice.net.
Key features
If you’re a tech enthusiast looking for innovation, Flipper Zero has some impressive key features that will catch your attention. First off, this multi-tool device combines versatility and convenience in one compact package. With its sleek design and ergonomic layout, it offers a user-friendly experience.
One of the standout features is its ability to emulate various types of RFID cards. This means you can clone access badges or transit cards with ease. Additionally, Flipper Zero comes equipped with an integrated infrared transmitter which allows you to control devices such as TVs and air conditioners effortlessly.
Moreover, the device supports multiple wireless communication protocols like Bluetooth Low Energy and NFC, making it compatible with a wide range of gadgets. These key features make Flipper Zero a game-changer in the world of tech tools, offering unparalleled functionality and flexibility for users.
Practical applications
Have you ever wondered how Flipper Zero’s innovative features can be applied in real-life scenarios? Let’s dive into some practical applications of this revolutionary multi-tool.
Imagine using Flipper Zero as a universal remote control to operate various electronic devices seamlessly. Whether it’s turning on your smart TV or adjusting the temperature on your air conditioner, Flipper Zero simplifies daily tasks with just a few clicks.
Additionally, for tech enthusiasts and hackers alike, Flipper Zero serves as a versatile tool for cybersecurity testing and auditing. Its ability to interact with RFID cards, clone access tokens, and emulate key fobs makes it an invaluable gadget for enhancing security protocols.
Moreover, in the realm of home automation, Flipper Zero can integrate effortlessly with smart home systems to manage lighting, appliances, and security systems remotely. With its programmable capabilities and user-friendly interface, the possibilities are endless when incorporating Flipper Zero into your tech-savvy lifestyle.
ZeroTier Networking Technology
ZeroTier Networking Technology is revolutionizing the way devices connect and communicate in today’s digital landscape. By leveraging a software-defined networking approach, ZeroTier enables seamless and secure peer-to-peer connections across any network infrastructure. This innovative technology eliminates the need for complex VPNs or expensive hardware solutions, making it an attractive option for businesses and individuals alike.
With ZeroTier, users can create virtual networks that span the globe with minimal setup time and effort. The decentralized nature of ZeroTier ensures robust security and privacy without compromising on performance. Whether you’re working remotely, collaborating with team members worldwide, or simply seeking a more efficient way to manage your devices, ZeroTier offers unparalleled flexibility and scalability.
By integrating ZeroTier technology with Flipper Zero, users can unlock even greater potential for remote access control and device management. Imagine being able to securely connect to your Flipper Zero from anywhere in the world with just a few clicks – that’s the power of ZeroTier at your fingertips!
Benefits of ZeroTier technology
ZeroTier technology offers numerous benefits in the realm of networking. One key advantage is its ability to create virtual local area networks (VLANs) across different devices and locations seamlessly. This means that users can easily connect and communicate with their devices regardless of physical distance, making remote work and collaboration more efficient.
Additionally, ZeroTier provides a secure network environment by encrypting all data transmissions end-to-end. This ensures that sensitive information remains protected from unauthorized access or cyber threats. Moreover, the technology is compatible with various operating systems, allowing for flexible integration across different platforms.
Furthermore, ZeroTier simplifies network management by centralizing control through a user-friendly interface. This streamlines configuration processes and enhances overall network performance. ZeroTier technology empowers users with a reliable and secure networking solution for modern connectivity needs.
Integration with Flipper Zero
The integration of Flipper Zero with various devices opens up a world of possibilities. By seamlessly connecting to smart gadgets, computers, or even IoT systems, Flipper Zero becomes a versatile tool in your tech arsenal.
Its compatibility with ZeroTier networking technology further enhances its capabilities by enabling secure and decentralized connections across different networks. This means you can control and monitor your devices remotely without compromising on security.
Whether it’s unlocking doors, emulating keycards, or interfacing with electronic locks, the applications are endless. The ability to customize the functionality of Flipper Zero through integrations allows for tailored solutions to fit your specific needs.
With this seamless integration approach, Flipper Zero truly stands out as a game-changer in the realm of multi-tool devices.
Latest Tech Advancements
Exciting advancements in technology are constantly shaping the world we live in. From artificial intelligence to quantum computing, the latest tech trends are pushing boundaries and opening up new possibilities. One notable innovation making waves is Flipper Zero – a revolutionary multi-tool designed to simplify digital security tasks.
With its compact design and versatile features, Flipper Zero offers a wide range of practical applications for cybersecurity professionals and tech enthusiasts alike. Whether it’s sniffing radio signals or emulating keycards, this all-in-one device is changing the game.
Another noteworthy development is ZeroTier networking technology, providing seamless connectivity across devices regardless of their physical location. By integrating ZeroTier with Flipper Zero, users can enhance their network security and streamline remote access operations.
In the fast-paced world of technology, staying updated on the latest advancements is crucial for both personal growth and professional success. Stay tuned for more cutting-edge innovations that are set to revolutionize the way we interact with technology.
Flipper Zero Documentation
Diving into the world of Flipper Zero documentation opens up a realm of possibilities for tech enthusiasts. The detailed guides provided offer a roadmap to navigate through the functionalities and capabilities of this innovative multi-tool. Whether you’re a novice or an experienced user, the documentation serves as a valuable resource to maximize your experience with Flipper Zero.
From setting up the device to exploring its various modes and features, each section of the documentation is meticulously crafted to provide clear instructions and insights. Troubleshooting tips are also included, ensuring that any issues encountered along the way can be swiftly resolved.
With step-by-step tutorials and visuals, navigating through the Flipper Zero documentation is intuitive and straightforward. It empowers users to unlock the full potential of this versatile tool and explore its endless possibilities in cybersecurity, hacking, RFID cloning, and more.
The comprehensive nature of the Flipper Zero documentation reflects the commitment to user satisfaction and support within Zerodevice.net’s ecosystem.
Conclusion
In the rapidly evolving tech landscape, staying up-to-date with the latest trends is crucial. Zerodevice.net serves as a hub for tech enthusiasts looking to explore cutting-edge innovations. From the revolutionary Flipper Zero multi-tool to the game-changing ZeroTier networking technology, there are endless possibilities to discover.
Flipper Zero offers a versatile solution with its key features and practical applications that cater to various needs in cybersecurity and IoT development. Its integration with ZeroTier further enhances its capabilities, enabling secure and seamless networking across devices.
With ongoing advancements showcased in Flipper Zero documentation, users can delve deeper into its functionalities and unleash its full potential. As technology continues to progress at a rapid pace, platforms like Zerodevice.net play a pivotal role in keeping us informed about the latest developments shaping our digital future.
Technology
Why Squarespace is Better than WordPress for SEO
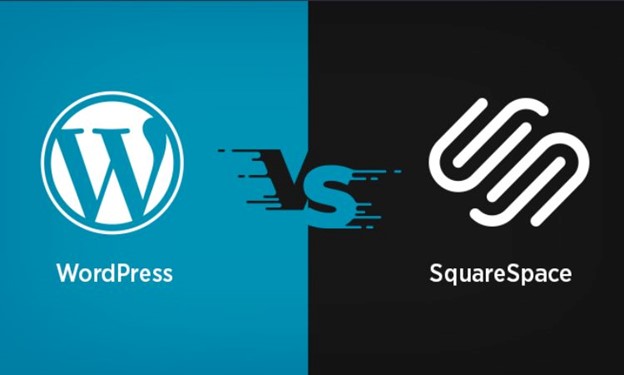
Are you trying to choose between Squarespace and WordPress as the best platform for your website? You’re not alone! It is one of the most asked questions by people who are in the business, running a blog or website, and everyone else who wants to make a presence on the internet. WordPress has always been considered the best SEO platform, but Squarespace has recently increased its efficiency significantly. But does Squarespace have all it takes to overcome WordPress, particularly in everything that has to do with search engine optimization?
The short answer? Absolutely. WordPress is highly customizable, and Squarespace has tools to help with SEO even when you don’t know much about it. It is better to have an “all-in-one” toolkit prepared and available instead of installing plugins and changing the code frequently.
When it comes to using Squarespace for SEO in 2025, you might find some new updates and features helpful. For instance, have you heard that Squarespace now automatically updates the schema markup with the latest features? Or does it come with built-in capabilities for image optimization for speed and SEO? But the usefulness of these features is in itself enough to save you tens of hours of work.
But we’re not here to bash WordPress. Instead, we’re going to jump right into why Squarespace could be a better solution for your SEO needs in 2025.
Key Takeaways:
- Squarespace’s built-in SEO features eliminate the need for additional plugins.
- Its automatic optimizations, like schema markup and lazy loading, save time and improve rankings.
- Squarespace focuses on speed and mobile compatibility, which are decisive factors for SEO in 2025.
- Unlike WordPress, Squarespace provides a simpler, streamlined experience for users with little to no technical knowledge.
Why Squarespace Beats WordPress for SEO
1. Built-In SEO Features
Squarespace allows you to avoid using numerous plugins and having a tech background to make the site efficient. Everything you need for SEO is already baked into the platform:
- Automatic XML Sitemaps: Squarespace automatically creates and updates a sitemap so that search engines can easily crawl your website.
- Customizable Page Titles and Descriptions: It is quite simple to update the metatags for each page and post and increase the probability of ranking for targeted keywords.
- Clean URLs: Unlike WordPress, Squarespace provides well-organized, clean, and SEO-friendly URLs for all pages by default.
This is unlike WordPress where you might need to install and set up plugins such as Yoast SEO or Rank Math to achieve such options.
2. Speed Optimization
The speed of the website is an important factor in ranking in 2025. Google states that any delay in the loading time of the page may lead to 11% fewer page views and 7% loss in conversions.
Squarespace speed optimization is easy as:
- It automatically compresses images without sacrificing quality.
- It employs pre-integrated Content Delivery Networks (CDNs) to deliver content faster to users all over the world.
- It provides a lazy loading feature for images to load them as soon as they are required to be loaded.
At the same time, WordPress can be slower if it is not optimized properly. Optimization involves selecting an appropriate hosting provider and installing caching and image compression plugins, which may be tiresome and error-prone. Similarly, users may need to take steps to speed up squarespace websites by optimizing images, reducing custom code, and leveraging built-in performance settings.
3. Mobile-First Design
In 2025, mobile-first indexing is expected to become normal for Google searches. This means that it is more important to get good performance on the mobile version of your site than on the desktop version. Squarespace templates are designed with a mobile-first approach, which means your site will look and work great on any device.
However, WordPress requires more effort when it comes to web development. You will have to select the theme and check its responsiveness, or else you will lose mobile traffic.
4. Automatic Schema Markup
Schema markup helps search engines better understand the content you provide and can result in enhanced snippets in the SERPs (star ratings, FAQ drop-downs, etc.). Squarespace incorporates schema markup into the site so that you can easily rank among other competitors.
For WordPress, using schema markup often requires a plugin, such as Schema or All in One Schema. That said, these plugins, though helpful, are additional in the sense that they come with more control and additional cost.
5. No Plugins Required
To be honest, WordPress needs a gazillion plugins and one cannot manipulate the site without having at least 5 installed. While plugins give you flexibility, they also come with risks:
- Incompatibility of the plugins can cause your site to become unresponsive or even crash.
- Plugins are known to drag your website speed if not well managed or optimized.
- Older plugins on your site can be potential security risks when not updated due to their various flaws.
Squarespace eliminates these issues. All of its SEO features are integrated, which means there is no need to worry about updates, compatibility issues, or extra charges.
6. User-Friendly Analytics
Tracking your site performance is important for improving your SEO strategy. Squarespace provides built-in analytics that are easy to understand, showing you metrics like:
- Search keywords driving traffic to your site.
- Which pages are performing best.
- Where your visitors are coming from.
WordPress does provide analytics through plugins such as Google Analytics, though the process can be somewhat complicated for beginners.
Unique Tips and Techniques for Squarespace SEO in 2025
- Leverage Built-In Blogging Tools: Blogs are great for SEO. With a simple blogging tool available on Squarespace, it is easy to create and publish quality articles which have your targeted keywords.
- Optimize Images Before Uploading: Originally, Squarespace automatically optimized images during uploading, but the quality of images will be even better if you prepare them as WebP size before uploading.
- Enable AMP for Blog Posts: AMP helps enhance the loading speed of mobile pages and increases the SEO rating of blog articles. Squarespace really simplifies the enabling of AMP as a feature, which can be done with one click.
- Focus on Local SEO: Squarespace allows businesses to use location-based schemas, which enhances their ability to rank for local search terms.
- Use Simple Navigation: Maintain the simplicity of your site’s navigation to enhance customers’ interaction and facilitate search engine crawling.
FAQs
Q: Is Squarespace better than WordPress for beginners?
A: Yes! Squarespace is much easier to use than WordPress, especially for first-time users who are not very familiar with the technicalities. Its main benefits are SEO-friendly features and the site’s simplicity.
Q: Can Squarespace handle complex SEO needs?
A: Although WordPress retains the edge in this area due to extra add-ons in technical SEO, Squarespace alone is already capable of providing enough tailored features for small to medium use.
Q: How do I track my SEO progress on Squarespace?
A: Squarespace comes equipped with all the necessary analytics one may want, including traffic and keywords.
Q: What about E-commerce SEO?
A: Squarespace is perfect for E-commerce SEO, especially when it comes to clean and optimized product links, schema markup, and fast-loading pages.
Conclusion
If you want to drop the complicated SEO procedures with no compromise on their effects, then Squarespace is the answer. Also, if you really want to rank and convert, improving your website’s speed is something you have to do. Whether you’re on Squarespace or considering switching, tools like Website Speedy tool can help you optimize your website speed, reduce bounce rates, and increase conversions.
Don’t beat around the bush—get your site moving, and start improving its speed now.
-
Articles10 months ago
How Many Times Can You Regrow Green Onions
-
News5 months ago
Understanding HotLeaks: What You Need to Know
-
Entertainment9 months ago
Bare it All: Unforgettable Skinny Dipping Stories Shared
-
Technology8 months ago
The Wonders of Oh Em Gee Blog
-
Fashion3 months ago
Opals in the USA: A Gemstone Transforming the Crystal Healing Market
-
Fashion9 months ago
Make a Statement with Stunning Black Homecoming Dresses
-
Health8 months ago
Can You Smoke Shrooms? Exploring the Myths and Realities
-
Articles4 days ago
WHAT IS THE DIFFERENCE BETWEEN SEED GARLIC AND FOOD GARLIC?