Technology
Data Analytics Architecture: A Comprehensive Glossary
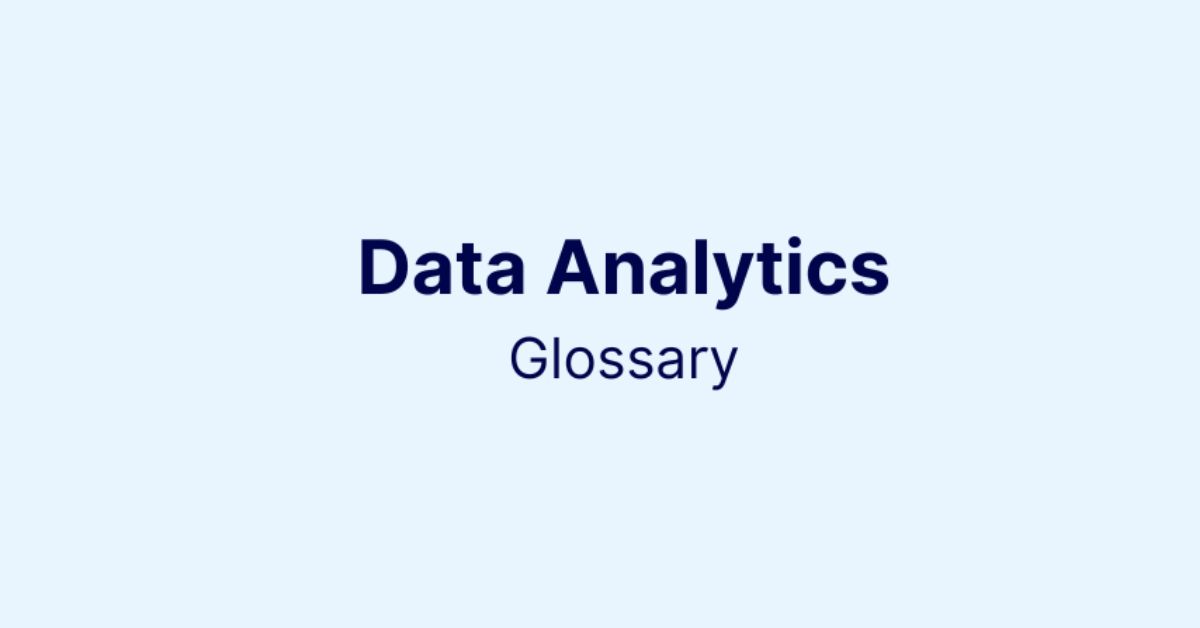
Data analytics has become the cornerstone of decision-making in the modern business environment. As organizations increasingly rely on data to guide their strategies, understanding the architecture that underpins data analytics is crucial. However, the terminology can be daunting, especially for those new to the field. This glossary aims to demystify the key terms and concepts in data analytics architecture, providing a clear and concise reference guide.
What is Data Analytics Architecture?
Data analytics architecture refers to the design and structure of systems that manage, process, and analyze large sets of data. It encompasses the tools, technologies, and methodologies used to collect, store, and interpret data, enabling organizations to derive insights and make informed decisions.
Key Components of Data Analytics Architecture
- Data Sources
Structured Data: Data that is organized in a fixed format, often in tables (e.g., databases).
Unstructured Data: Data that does not have a predefined structure (e.g., text, images).- Semi-Structured Data: Data that does not fit into a rigid structure but has some organizational properties (e.g., JSON, XML files).
- Data Ingestion
Batch Processing: Collecting and processing data in large volumes at specified intervals.- Stream Processing: Real-time data processing as it flows into the system.
- Data Storage
Data Warehouse: Centralized repository for structured data, optimized for querying and reporting.
Data Lake: A storage system that holds large volumes of raw, unprocessed data in its native format.- NoSQL Databases: Databases designed to handle a variety of data types, including unstructured and semi-structured data.
- Data Processing
ETL (Extract, Transform, Load): A process that involves extracting data from sources, transforming it to a usable format, and loading it into a storage system.- ELT (Extract, Load, Transform): Similar to ETL, but data is first loaded into the storage system and then transformed.
- Data Analysis
Descriptive Analytics: Analysis that describes historical data and trends.
Predictive Analytics: Analysis that uses historical data to predict future outcomes.- Prescriptive Analytics: Analysis that recommends actions based on data insights.
- Data Visualization
Dashboards: Visual representations of data that provide insights at a glance.
Reports: Detailed data presentations, often used for decision-making.- Data Mining: The process of discovering patterns and relationships in large datasets.
- Data Governance
Data Quality Management: Ensuring data is accurate, consistent, and reliable.
Data Privacy: Protecting sensitive data from unauthorized access.- Data Compliance: Adhering to legal and regulatory requirements for data management.
Glossary of Essential Data Analytics Architecture Terms
A. Data Sources
- APIs (Application Programming Interfaces): A set of protocols for building and interacting with software applications, enabling the exchange of data between systems.
- Data Feeds: Continuous streams of data from external sources, such as social media, stock market data, or weather reports.
- Data Pipelines: A series of data processing steps that move data from one system to another.
B. Data Ingestion
- Data Lake Ingestion: The process of moving data from its source into a data lake.
- Change Data Capture (CDC): A technique used to identify and capture changes in data so they can be applied to other systems.
- Message Queues: A form of asynchronous service-to-service communication used in serverless and microservices architectures.
C. Data Storage
- Columnar Databases: Databases that store data in columns rather than rows, optimized for reading and querying large datasets.
- Distributed File Systems: Systems that manage data across multiple storage devices, enabling large-scale data storage and access.
- In-Memory Databases: Databases that store data in the main memory of a computer, allowing for extremely fast data retrieval.
D. Data Processing
- Data Orchestration: The automated coordination and management of data workflows and processes.
- Data Transformation: The process of converting data from one format or structure to another.
- Big Data Processing Frameworks: Tools like Apache Hadoop and Apache Spark that enable the processing of large datasets across distributed systems.
E. Data Analysis
- Machine Learning Algorithms: Algorithms that enable computers to learn from data and make predictions or decisions without being explicitly programmed.
- Natural Language Processing (NLP): A branch of AI that helps machines understand and interpret human language.
- Data Marts: Subsets of data warehouses focused on specific business areas, such as sales or finance.
F. Data Visualization
- Geospatial Visualization: Visualization of data that includes a geographical component, often using maps.
- Heatmaps: Visual representations of data where individual values are represented by colors.
- Time-Series Analysis: Analyzing data points collected or recorded at specific time intervals.
G. Data Governance
- Data Stewardship: The management and oversight of an organization’s data assets to ensure data quality and compliance.
- Metadata Management: The administration of data that describes other data, providing context and meaning.
- Data Lineage: The tracking of data’s origin, movements, and transformations across the system.
Challenges in Data Analytics Architecture
- Scalability
- As data volumes grow, the architecture must scale to accommodate the increased load without sacrificing performance.
- Data Integration
- Combining data from diverse sources and formats into a unified view can be complex and time-consuming.
- Real-Time Processing
- Achieving low-latency data processing to provide insights in real-time is challenging but essential for many applications.
- Security and Privacy
- Protecting data from breaches and ensuring compliance with regulations like GDPR are critical concerns.
Best Practices for Designing Data Analytics Architecture
- Start with a Clear Strategy
- Define the goals and requirements of your data analytics initiatives before designing the architecture.
- Use Modular Design
- Implement a modular architecture that allows for flexibility and scalability as needs evolve.
- Prioritize Data Governance
- Establish strong data governance practices to ensure data quality, security, and compliance.
- Leverage Automation
- Automate data processes where possible to reduce manual effort and improve efficiency.
- Choose the Right Tools
- Select tools and technologies that align with your organization’s needs and capabilities.
Conclusion
Understanding the intricacies of data analytics architecture is essential for any organization looking to harness the power of data. By familiarizing yourself with the key terms and concepts outlined in this glossary, you’ll be better equipped to navigate the complexities of data analytics and drive more informed decision-making.
FAQs
- What is the difference between a data warehouse and a data lake?
- A data warehouse is designed for storing structured data and is optimized for querying and reporting. In contrast, a data lake stores raw, unprocessed data in its native format, making it suitable for storing a wide variety of data types.
- Why is data governance important in data analytics architecture?
- Data governance ensures that data is accurate, secure, and compliant with regulations, which is crucial for maintaining trust and integrity in data-driven decision-making.
- What are some common challenges in building a data analytics architecture?
- Common challenges include scalability, data integration, real-time processing, and ensuring data security and privacy.
- How does ETL differ from ELT in data processing?
- ETL involves extracting data, transforming it, and then loading it into storage, while ELT loads data first and then performs the transformation within the storage system.
- What role does machine learning play in data analytics?
- Machine learning algorithms analyze data to identify patterns, make predictions, and automate decision-making processes, enhancing the value of data analytics.
Technology
Jacksonville Computer Network Issues: Problems & Practical Fixes
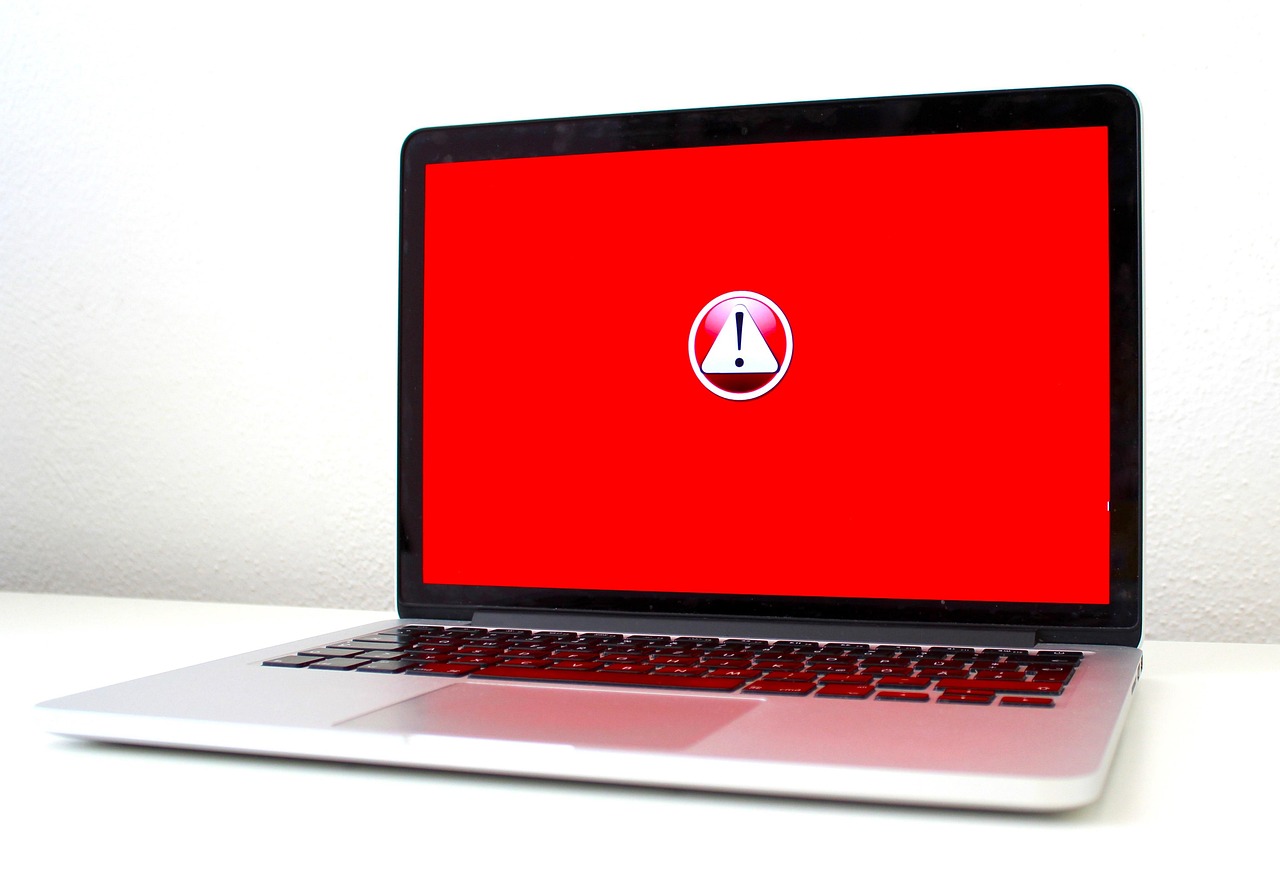
Computer networks are the backbone of modern businesses in Jacksonville, ensuring seamless communication, data sharing, and operational efficiency. However, network issues can disrupt productivity, leading to downtime, security risks, and financial losses. Whether you’re a business owner, IT professional, or a concerned user, understanding common Jacksonville computer network issues—and how to resolve them—can save time and frustration.
In this guide, we’ll explore the most frequent network problems in Jacksonville, their causes, and actionable solutions to keep your systems running smoothly.
Common Jacksonville Computer Network Issues
1. Slow Network Performance
A sluggish network is one of the most frustrating issues for businesses and home users alike. Slow speeds can stem from:
-
Bandwidth congestion – Too many devices consuming data simultaneously.
-
Outdated hardware – Old routers, switches, or cables limiting performance.
-
ISP throttling – Some providers slow down speeds during peak hours.
-
Malware or viruses – Hidden infections consuming network resources.
How to Fix It:
✔ Upgrade hardware – Invest in modern routers and switches.
✔ Limit bandwidth-heavy activities – Prioritize critical tasks.
✔ Run antivirus scans – Remove malware affecting performance.
✔ Contact your ISP – Ensure you’re getting the promised speeds.
2. Intermittent Connectivity (Dropped Connections)
Frequent disconnections disrupt remote work, VoIP calls, and online transactions. Causes include:
-
Faulty cables or ports – Damaged Ethernet cables or router ports.
-
Wi-Fi interference – Nearby electronics (microwaves, Bluetooth devices).
-
ISP instability – Poor infrastructure or maintenance issues.
How to Fix It:
✔ Check physical connections – Replace damaged cables.
✔ Change Wi-Fi channels – Use tools like Wi-Fi Analyzer to find less congested channels.
✔ Reset network devices – Power cycle modems and routers.
✔ Consider a wired connection – Ethernet offers more stability than Wi-Fi.
3. DNS Resolution Problems
When websites won’t load but other internet services work, DNS issues may be the culprit. Symptoms include:
-
“DNS Server Not Responding” errors
-
Slow website access despite good speed tests
How to Fix It:
✔ Switch to a reliable DNS server (Google DNS: 8.8.8.8
or Cloudflare: 1.1.1.1
).
✔ Flush DNS cache – Run ipconfig /flushdns
in Command Prompt.
✔ Restart your router – Resets DNS configurations.
4. IP Address Conflicts
When two devices share the same IP, connectivity fails. This often happens in offices with static IP mismanagement.
How to Fix It:
✔ Use DHCP – Let the router assign IPs automatically.
✔ Manually assign unique IPs – For devices needing static IPs.
✔ Restart affected devices – Refreshes IP assignments.
5. Security Vulnerabilities & Cyber Threats
Jacksonville businesses face increasing cyber risks, including:
-
Phishing attacks – Fake emails stealing credentials.
-
Ransomware – Encrypting files for ransom.
-
Unauthorized access – Weak passwords exposing networks.
How to Fix It:
✔ Install firewalls & antivirus software – Blocks malicious traffic.
✔ Enable multi-factor authentication (MFA) – Adds extra security.
✔ Train employees – Teach them to recognize phishing scams.
✔ Regularly update software – Patches security flaws.
Proactive Measures to Prevent Network Issues
1. Regular Network Maintenance
-
Monitor performance – Use tools like PingPlotter or SolarWinds.
-
Update firmware – Keeps routers and switches secure.
2. Invest in Quality Hardware
-
Enterprise-grade routers – Better for businesses than consumer models.
-
Uninterruptible Power Supply (UPS) – Prevents downtime during outages.
3. Backup Critical Data
-
Cloud backups – Protects against ransomware.
-
Local backups – Ensures quick recovery.
4. Work with IT Professionals
For complex issues, Jacksonville-based IT support can provide:
-
Network assessments – Identifies vulnerabilities.
-
24/7 monitoring – Prevents major outages.
Final Thoughts
Jacksonville computer network issues can disrupt productivity, but with the right knowledge and tools, most problems are fixable. By understanding common issues—slow speeds, dropped connections, DNS errors, and security risks—you can take proactive steps to maintain a stable and secure network.
For businesses, partnering with a reliable IT service ensures long-term network health. Stay updated, invest in quality infrastructure, and train employees to minimize risks.
Technology
Unveiling the World of Snuffers: A Comprehensive Guide
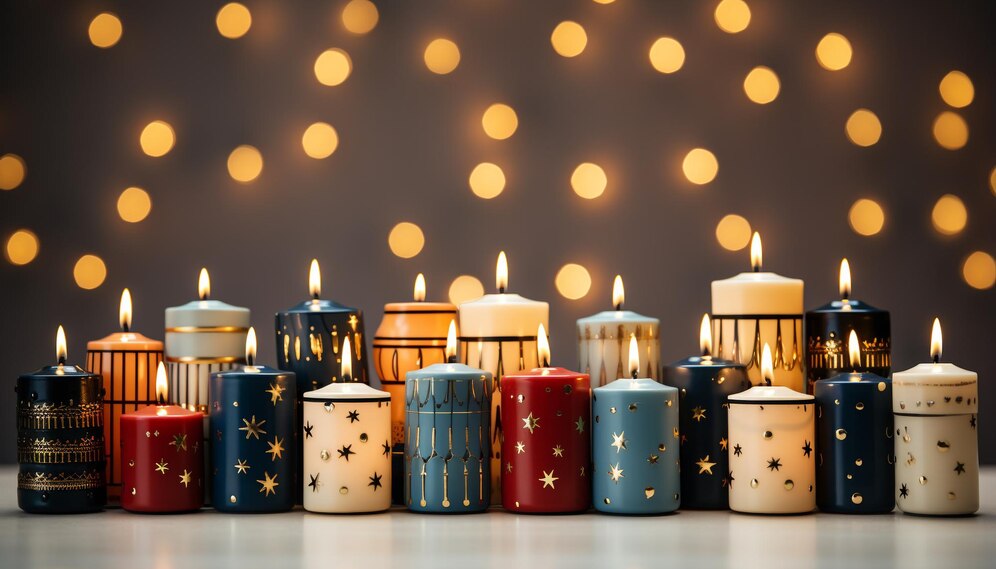
Welcome to the enchanting world of snuffers, where practicality meets elegance in the art of extinguishing candles. Whether you’re a candle enthusiast or simply looking to add a touch of sophistication to your decor, snuffers are here to elevate your candle experience. Join us on a journey as we unveil the beauty and functionality of different types of snuffers, from classic designs to modern innovations. Let’s dive in and explore the fascinating realm of these essential candle accessories!
Beehive Candle Snuffer
The Beehive Candle Snuffer is a charming and practical accessory for any candle lover. Its unique beehive shape adds a whimsical touch to your decor while serving the important function of safely extinguishing candles. Crafted from high-quality materials, this snuffer ensures a clean and efficient way to put out candles without the need for blowing, preventing wax splatters and preserving the wick for future use. Embrace both style and functionality with the Beehive Candle Snuffer, a must-have tool for every candle enthusiast’s collection.
Description
Embark on a journey to discover the world of snuffers, an essential tool for any candle enthusiast. A snuffer is a device used to extinguish candles safely and efficiently without causing smoke or hot wax splatters. With various designs available, such as the Beehive Candle Snuffer, each snuffer brings its unique charm to your candle-lit moments. From traditional metal options to modern silicone alternatives, there’s a snuffer for every style preference. Delve into the intricate details of these fascinating accessories that add both practicality and elegance to your home decor setup.
Related products
When exploring the world of snuffers, it’s essential to consider related products that can enhance your candle extinguishing experience. From elegant Beehive Candle Snuffers to innovative Dragon Egg Snuffers, there are various options available to suit different preferences and styles. These related products not only provide practical solutions for safely putting out candles but also add a touch of sophistication and charm to your space. Experiment with different designs and materials to find the perfect complement for your candle collection.
Whether you opt for a traditional Taper Candle Snuffer or a modern Silicone Snuffer, each product offers unique features that cater to diverse needs. Discover how these related products can elevate your candle rituals and make extinguishing flames effortless yet stylish.
Snuffers
Welcome to the world of snuffers! These handy tools are a must-have for any candle enthusiast. Snuffers come in various shapes and sizes, each designed to help you safely extinguish candles without the mess or hassle of blowing them out.
Whether you prefer a classic taper candle snuffer or a modern silicone snuffer, there’s something for everyone. Discover the convenience and elegance that snuffers bring to your candle-lit moments.
Dragon Egg Snuffer
Looking for a touch of whimsy in your candle accessories? Enter the Dragon Egg Snuffer, a unique and enchanting tool that adds a mystical flair to extinguishing candles. With its intricate dragon egg design, this snuffer is not just functional but also serves as a decorative piece for any room.
Crafted with attention to detail, the Dragon Egg Snuffer brings an element of fantasy into your daily routine. Say goodbye to mundane snuffers and embrace the magic of this intriguing accessory for your candle collection.
Silicone Snuffer
Have you heard about silicone snuffers? These innovative tools are designed to safely extinguish candles without the risk of hot wax splatter. Silicone snuffers are heat-resistant and can be easily cleaned, making them a practical choice for candle enthusiasts.
Their flexible and soft material allows for gentle extinguishing, ensuring that your candles stay intact and free from damage. With various colors and designs available, silicone snuffers not only serve a functional purpose but also add a touch of style to your candle accessories collection.
Why use a silicone snuffer?
Silicone snuffers are the modern solution for safely extinguishing candles without the risk of hot wax splattering. The flexible and heat-resistant material makes them durable and easy to use, ensuring a clean and efficient candle-snuffing experience.
Their non-abrasive nature also protects delicate candle wicks from damage, prolonging the life of your favorite candles. Say goodbye to traditional metal snuffers that can sometimes cause marks or scratches on your candles—switch to silicone for a hassle-free way to keep your ambiance intact.
Specifications
When it comes to snuffers, understanding the specifications is key. The materials used, dimensions, and design details can all play a role in how effective a snuffer will be for your candle extinguishing needs.
Silicone snuffers are lightweight and heat-resistant, making them safe and easy to use. With a long handle for reaching candles in deep holders, they offer convenience and versatility. Paying attention to these specifications ensures you find the perfect snuffer for your candle care routine.
Related products
When it comes to snuffers, there are various related products that can enhance your candle-snuffing experience. From classic designs to innovative options, there is a wide range of choices available for every preference. Whether you prefer the traditional elegance of a beehive candle snuffer or the sleek functionality of a silicone snuffer, there is something out there for everyone.
For those looking for a unique touch, dragon egg snuffers offer a whimsical twist on a practical tool. Additionally, taper candle snuffers provide precision when extinguishing smaller candles with ease and finesse. Explore these related products to find the perfect companion for your candle care routine.
Taper Candle Snuffer
The Taper Candle Snuffer is a handy tool for extinguishing candles without the mess of blowing them out. With its long handle and cone-shaped end, it allows you to reach candles in tall holders or deep jars easily. Simply place the snuffer over the flame, cutting off its oxygen supply and putting out the candle with minimal smoke or splatter.
Using a Taper Candle Snuffer not only adds an elegant touch to your candle ritual but also helps prolong the life of your candles by preventing wicks from getting charred. Say goodbye to smoky rooms and wax splatters – invest in a Taper Candle Snuffer for a cleaner and more sophisticated candle experience!
Candle Snuffer
The candle snuffer is a practical tool designed to extinguish candles safely and effectively. Its long handle allows users to reach into deep or narrow candle holders without the risk of burns. By simply placing the bell-shaped end over the flame, you can quickly put out the candle without any mess or smoke.
Historically, candle snuffers were popular in households where candles were the primary source of lighting. Today, they remain a handy accessory for anyone looking to prolong their candle’s lifespan and avoid unnecessary spills or smoky odors in their living space.
Description
Snuffers are essential tools for safely extinguishing candles without the risk of hot wax splatters. Among the variety of snuffers available, the Beehive Candle Snuffer stands out with its elegant design and practical functionality. Crafted from high-quality materials, this snuffer features a long handle for easy reach and a bell-shaped top to efficiently smother flames. Whether you’re a candle enthusiast or simply enjoy creating a cozy ambiance at home, the Beehive Candle Snuffer is a must-have accessory that combines style with utility.
Historical usage
In the intriguing world of snuffers, historical usage adds a touch of nostalgia and tradition. Back in the day, before modern conveniences like lighters and electric candles, snuffers were essential tools for extinguishing flames. Their long handles allowed individuals to safely reach and put out candles without risking burns or accidents.
Throughout history, snuffers were not only practical but also considered elegant accessories that adorned households with sophistication. From grand castles to humble abodes, these tools played a vital role in maintaining candlelit spaces while showcasing exquisite craftsmanship.
Conclusion
Snuffers are essential tools for safely extinguishing candles without the risk of hot wax splatter or accidental fires. Whether you opt for a traditional metal snuffer like the beehive candle snuffer, a whimsical dragon egg snuffer, or a modern silicone option, each type offers its unique advantages in candle care and maintenance.
By investing in a high-quality snuffer and incorporating it into your candle routine, you can enhance both the safety and longevity of your favorite candles while adding an elegant touch to your decor. So why not explore the world of snuffers today and elevate your candle experience to new heights?
Technology
SERPClix vs SERPSEO: Which is Best for Your SEO Strategy?
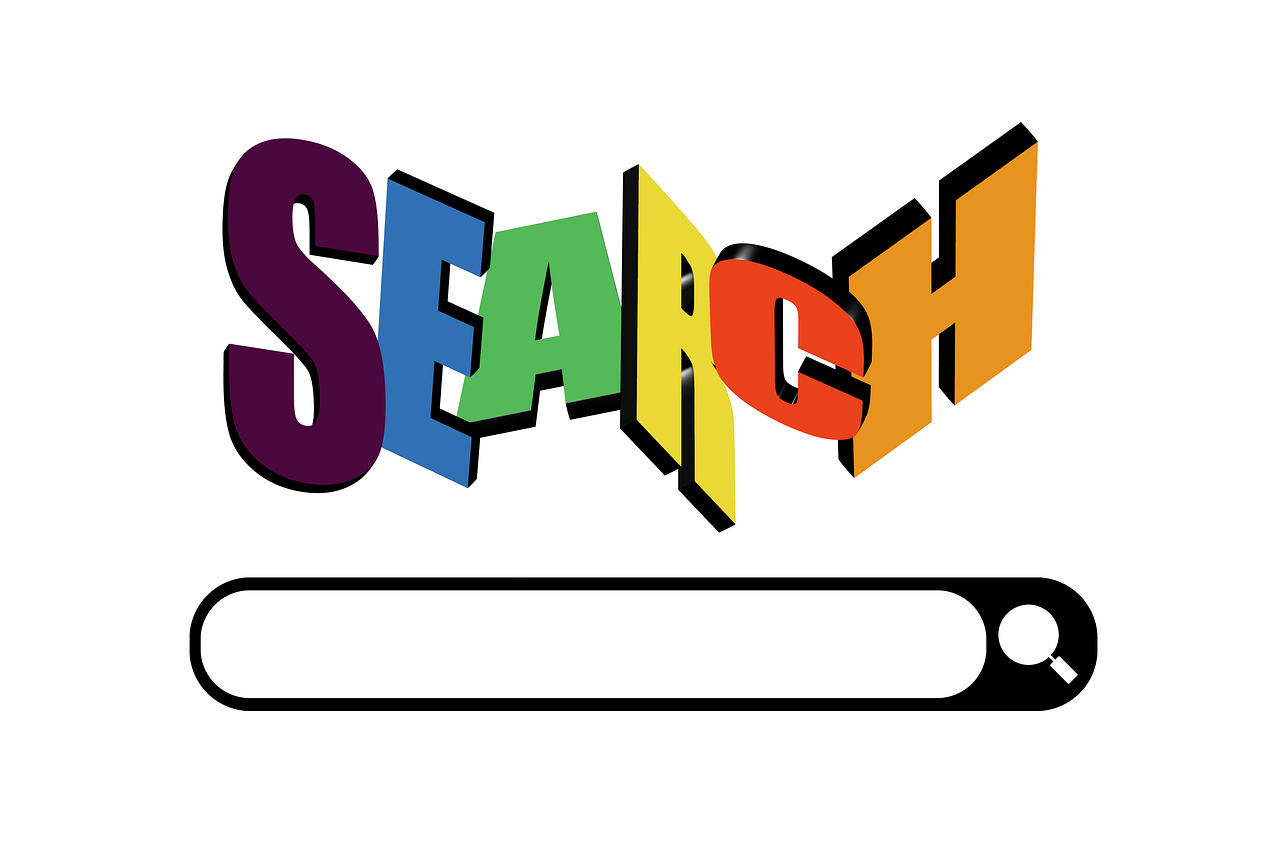
In the competitive world of SEO, tools like SERPClix and SERPSEO have gained attention for their ability to improve search engine rankings. Both platforms offer unique features designed to boost organic traffic, but which one is right for you?
This in-depth comparison will explore the key differences, pros, cons, pricing, and effectiveness of SERPClix and SERPSEO. By the end, you’ll have a clear understanding of which tool aligns best with your SEO goals.
What is SERPClix?
SERPClix is a pay-per-click (PPC) platform that helps improve keyword rankings by simulating real user searches and clicks. It works by having real users search for your target keywords, click on your website, and stay for a short duration—mimicking organic traffic behavior.
Key Features of SERPClix
-
Manual Clicks by Real Users – Ensures authenticity.
-
Supports Multiple Search Engines – Works with Google, Bing, and Yahoo.
-
Affordable Pricing – Cost-effective for small businesses.
-
No Bot Traffic – Complies with search engine guidelines.
Pros of SERPClix
✅ Improves CTR (Click-Through Rate) – Higher CTR signals relevance to Google.
✅ Safe & White-Hat Friendly – Avoids penalties by using real users.
✅ Easy to Use – Simple dashboard and setup process.
Cons of SERPClix
❌ Limited Automation – Requires manual keyword submissions.
❌ Slower Results – Takes time to accumulate clicks.
❌ No Advanced Analytics – Lacks in-depth performance tracking.
What is SERPSEO?
SERPSEO is an automated SEO tool designed to enhance search rankings through AI-driven traffic generation, backlink building, and keyword optimization. Unlike SERPClix, it focuses on automated engagement signals to improve rankings.
Key Features of SERPSEO
-
AI-Powered Traffic Simulation – Mimics real user behavior.
-
Automated Backlinks – Builds high-authority links.
-
Keyword & Rank Tracking – Monitors SERP movements.
-
Fast Results – Delivers quicker ranking improvements.
Pros of SERPSEO
✅ Faster Ranking Improvements – AI accelerates results.
✅ Comprehensive SEO Suite – Includes backlinks, traffic, and analytics.
✅ Automated Process – Saves time compared to manual methods.
Cons of SERPSEO
❌ Risk of Over-Optimization – Potential for unnatural traffic spikes.
❌ Higher Cost – More expensive than SERPClix.
❌ Learning Curve – Requires some SEO knowledge to optimize.
SERPClix vs SERPSEO: Head-to-Head Comparison
Feature | SERPClix | SERPSEO |
---|---|---|
Traffic Type | Manual (Real Users) | AI-Generated |
Speed of Results | Slow & Steady | Fast |
Pricing | Affordable | Premium |
Automation Level | Low | High |
Best For | Small Businesses, Beginners | Agencies, Advanced Users |
Risk of Penalty | Low | Moderate |
CTR Improvement | Strong | Moderate |
Backlink Support | No | Yes |
Which One Should You Choose?
Choose SERPClix If:
✔ You want a low-risk, manual approach to improve CTR.
✔ You’re on a budget and need gradual ranking improvements.
✔ You prefer white-hat SEO techniques without automation.
Choose SERPSEO If:
✔ You need faster rankings with AI-powered automation.
✔ You want an all-in-one SEO tool (traffic + backlinks).
✔ You’re comfortable with moderate risk for quicker results.
Best Practices for Using SERPClix & SERPSEO
-
Combine Both for Maximum Impact – Use SERPClix for CTR and SERPSEO for backlinks.
-
Monitor Rankings Regularly – Track progress to adjust strategies.
-
Avoid Overuse – Stick to natural-looking traffic patterns.
-
Focus on Quality Content – SEO tools work best with strong on-page optimization.
Final Verdict: Which is Better?
Both SERPClix and SERPSEO serve different purposes in SEO:
-
SERPClix is ideal for organic CTR improvement with minimal risk.
-
SERPSEO is better for fast rankings with AI automation.
If you prioritize safety and gradual growth, go with SERPClix. If you need quick results with automation, SERPSEO is the better choice.
For the best outcome, consider using both strategically—boosting CTR with SERPClix while leveraging SERPSEO for backlinks and AI-driven traffic.
Conclusion
Choosing between SERPClix vs SERPSEO depends on your SEO goals, budget, and risk tolerance. While SERPClix offers a manual, safe approach, SERPSEO provides automated, faster results.
By understanding their strengths and weaknesses, you can make an informed decision to enhance your search rankings effectively.
Which one will you try first? Let us know in the comments!
-
Articles1 month ago
How Many Times Can You Regrow Green Onions
-
News9 months ago
Understanding HotLeaks: What You Need to Know
-
Fashion6 months ago
Opals in the USA: A Gemstone Transforming the Crystal Healing Market
-
Technology12 months ago
The Wonders of Oh Em Gee Blog
-
Entertainment12 months ago
Bare it All: Unforgettable Skinny Dipping Stories Shared
-
Entertainment6 months ago
How to Use Snaptik: A Complete Guide to Download TikTok Videos
-
Health12 months ago
Can You Smoke Shrooms? Exploring the Myths and Realities
-
Articles4 months ago
WHAT IS THE DIFFERENCE BETWEEN SEED GARLIC AND FOOD GARLIC?